Right now, we’re at the infancy in the development of a whole new layer of added business value. AI and especially deep learning have tremendous potential to improve network planning and operations. You can get dizzy thinking about how much data is collected and monitored each day in networks across the world, and yet the vast majority of it goes ignored. It’s only when some network failure occurs that we dig into our pile of data to understand what happened.
That is a backward-looking application of data. Of course, it’s an important one – when we understand how failures happened, we can try to safeguard against similar incidents in the future. But avoiding failures in the first place is much better, and this is the promise of predictive maintenance. That is a forward-looking application of data and also the one that is garnering the most interest from network operators.
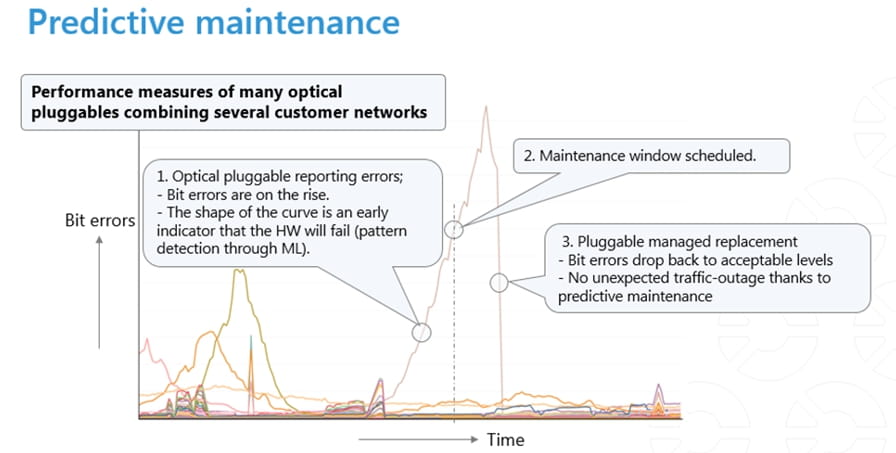
Predictive maintenance – today and in the near future
Equipment manufacturers such as our team at ADVA are starting to develop predictive maintenance functionality, and we’re excited about our progress. Our first proof of concept was to develop an AI model that could recognize a failure signature from a particular device and be robust enough to avoid triggering false positives.
The AI model has run for several months now and has performed very well. This is one simple example of AI learning to recognize early warning signs of a likely equipment failure. Because it’s a well-bounded problem we could achieve human-level performance with a relatively small data set.
In the near future we’ll develop the ability to predict not only equipment failures at the node level, but also link-level and network-level troubles. That means learning and correlating from data collected across the network, not just from a single node. The problem moves from a well-bounded definition to a complex and unpredictable space. This is the home ground of neural networks and deep learning.
Deep learning as an approach feels right but there are obstacles – and the biggest one facing all of us is getting enough data to train our algorithms. It’s well-known that AI algorithms are only as good as the data they learn from, and approaches such as deep learning can be helpful in solving complex and nuanced problems as long as we have a lot of high quality data available. Lots of data. And domain knowledge about networking. Deep domain knowledge.
It has to be a partnership
So the two ingredients of data and domain knowledge have to come together in order for network analytics to advance.
The best domain knowledge about a particular piece of equipment resides with the equipment manufacturers themselves. Not every network interface behaves identically, and indeed the specific variances and tradeoffs between vendors is part of the competitive secret sauce. However, our data sets are deep but narrow. We know a lot of detail about how our equipment works, but when it comes to data on network-level behavior there’s no better place to look than network operators.
When you consider that the key to network analytics is made of two halves, it’s obvious that a partnership between network operators and equipment manufacturers will be the enabling factor in driving network analytics forward.
What can equipment manufacturers do?
Equipment manufacturers must make it easy for network operators to safely and privately share data without violating legal or corporate security requirements. Customers will share data when the value outweighs the perceived risk. The value is already high, and it’s up to us to lower the risk. For ADVA it means we store everything we collect within our premises, on the same IT infrastructure which we use for our own business data. We have skin in the game when we treat customer data with the same care as our own. Our transfer mechanism is a push-model, where customers can push data securely into ADVA’s storage area at their own pace (as opposed to ADVA pulling data whenever we want). Our customers can start and stop data collection at any time and we feel it’s important that we put the ownership of data distribution into the hands of our customers.
What can network operators do?
On the network operator side, it will be necessary to review the existing security protocols against the new connected world we live in. Transport networks have traditionally been segregated areas with no direct access to the outside world. That is both a nice security feature and an obstacle to predictive maintenance. Network appliances will include ways to send performance data home, and the challenge is to find a way to let that happen without compromising network security.
Next, find an equipment vendor to work with, and start forming partnerships. We’re not at a point where anyone feels comfortable publishing data sets in the public domain, so a more pragmatic approach is to work with a trusted vendor where both vendor and operator have control over what happens to the data.
Data sharing does not have to mean an open firewall with streaming telemetry, and it doesn’t have to mean real-time. Network equipment already deals with real-time events such as fiber cuts and equipment failures, and that won’t go away. Network analytics deals with problems on a wider scale and longer timelines. We can do a lot with once-a-day or once-a-week data transfers via SFTP. It’s encouraging that we’re working with several network operators that understand that network performance data is largely anonymous and useless to an uninvited third party, so we’re able to anonymize and exchange data.
Moving forward
The ability to collect performance data is becoming more and more a value-added function, and our industry will eventually find a way to open up. After all, if Rolls Royce has found a way to stream real-time telemetry from their jet engines in-flight, then I’m confident that we’ll find ways to securely connect network appliances to their respective equipment manufacturers.